Insights Into Data Science: Lessons From 50 Articles
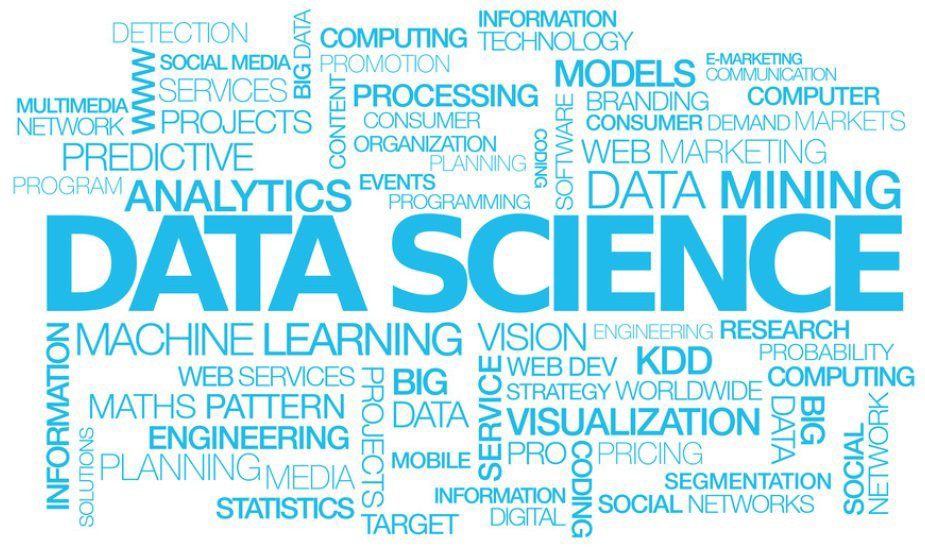
Insights Into Data Science
The ever-evolving field of data science offers countless lessons for professionals and enthusiasts alike. In a reflective series, Malaya Rout, Director of Data Science at Exafluence, distilled insights from 50 of his articles into a single piece of wisdom per article. These insights encompass foundational principles, advanced AI concepts, and practical applications, providing a roadmap for navigating the complex data science landscape.
Data science’s transformative power is undeniable, but the road to mastering it is filled with challenges. To explore how data analytics shapes industries, check out our feature on the growing importance of big data in business.
Key Takeaways From 50 Articles
- The Foundation of AI and LLMs: Large Language Models (LLMs) rely on content from original creators who often receive neither credit nor compensation.
- Navigating AI Projects: Many AIML projects fail to meet expected business outcomes due to obstacles in execution.
- The Value of Context: LLMs can be contextualized using fine-tuning, Retrieval-Augmented Generation (RAG), or both.
- Strategic Approach: A well-defined data strategy is critical for leveraging Generative AI effectively.
For a deeper understanding of data strategy applications, explore breaking into a business analytics career.
- Human-Centric Design: Even in B2B scenarios, viewing the customer as a person, not just an entity, is essential.
- Essential Skill Set: Data scientists require a blend of technical expertise, domain knowledge, soft skills, and creativity.
- Challenges in Modeling: Real-life complexities often resist complete representation through variables.
- Generative AI Limitations: Generative AI offers the illusion of understanding language but lacks true comprehension.
For a closer look at AI’s role in climate solutions, check out our article on data science and climate change mitigation.
Learning Through Innovation
Malaya Rout emphasizes that success in data science requires a blend of curiosity and practical thinking. From learning fundamental techniques like data exploration to advanced methods like multi-agent LLM architecture, the pathway to mastery involves persistence.
Key technical insights include:
- Scaling numeric variables is crucial for maintaining model accuracy.
- The Central Limit Theorem (CLT) is fundamental to machine learning.
- Fine-tuning LLMs enhances their value far beyond out-of-the-box capabilities.
For those seeking career clarity, our article on top data science career questions offers guidance.
The Future of Data Science
As AI-powered tools become omnipresent, their impact extends across industries. LLM-based applications, for instance, are poised to revolutionize workflows. However, caution is needed to avoid over-reliance on AI pair programmers, which may hinder long-term skill development.
Generative AI, despite its transformative potential, must be adopted judiciously. For businesses and developers, integrating Human-in-the-Loop (HITL) checkpoints ensures that models deliver reliable outcomes.
Explore more about these evolving trends in The Times of India’s comprehensive blog on data science innovations.
The journey of mastering data science is as much about learning from failures as it is about building technical expertise. Whether it’s understanding LLMs, navigating AIML project pitfalls, or embracing generative AI innovations, the insights from Malaya Rout’s articles offer invaluable lessons.
As the field continues to grow, staying informed and adaptable will remain critical for success.