Generative AI tools are revolutionizing creativity and education, producing millions of images daily. But despite their impressive capabilities, these tools often reflect the biases inherent in their training datasets, perpetuating outdated stereotypes. A recent study published in the Journal of Chemical Education highlights these biases, revealing how generative AI inaccurately portrays the diversity of chemists in gender, race, and disability representation.
What Does a Chemist Look Like?
For decades, the question “What does a scientist look like?” has yielded stereotypical answers—most often male, White, and wearing a lab coat. However, evolving social attitudes have led children today to draw more diverse scientists, including women and people of color. This shift prompts a critical question: are AI image generators keeping pace with these changing perceptions?
Researchers led by Valeria Stepanova, Meagan Kaufenberg-Lashua, Joseph West, and Jaime Kelly decided to investigate this question by prompting four AI image-generation models to create modern portraits of chemists in academic and industrial settings.
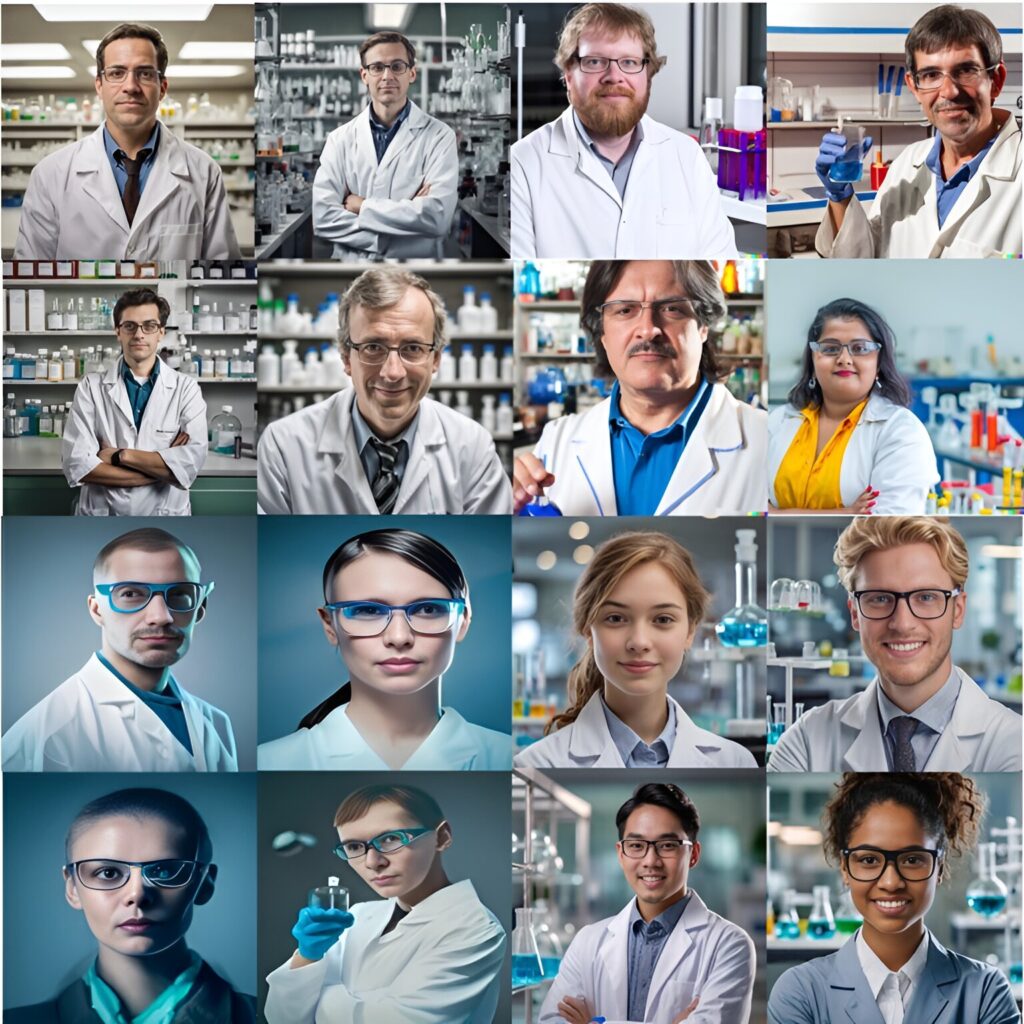
AI’s Missed Potential for Diversity
The study analyzed 200 AI-generated images to assess how well these tools reflected real-world demographics. While some results aligned with the U.S. National Science Foundation’s (NSF) 2021 survey data, discrepancies in gender, race, and disability representation were significant.
Key Findings:
- Gender Representation:
The overall male-to-female ratio of generated chemists was similar to NSF data. However, individual models varied significantly, with one producing only male images and another overrepresenting women. - Racial Representation:
Most images depicted White individuals, reflecting the U.S. chemistry field’s current demographics. Yet, two models generated almost no people of color, while one predominantly featured people of color. - Disability Representation:
None of the models produced images of chemists with visible disabilities, exposing a glaring gap in representation.
Such inconsistencies highlight how generative AI tools amplify biases and fail to depict the full diversity of professionals in STEM fields.
For insights into how generative AI aids predictive AI tasks, read here.
Why Representation in AI Matters
Biases in generative AI reflect the limitations of training datasets. These tools are only as good as the data they learn from, which often mirrors historical inequalities. When it comes to representing occupations like chemistry, these biases could have lasting effects on public perception and career aspirations.
AI images influence how future generations visualize professions. If these tools perpetuate outdated stereotypes, they risk undermining decades of progress toward inclusivity. As the researchers aptly ask, “Will humans control the knowledge generated by AI, or will AI shape how future generations perceive the world?”
Learn about AI scaling laws and their diminishing returns to better understand the limits of these technologies.
Addressing Bias in Generative AI
The study underscores the need for more inclusive AI development practices. Companies must curate diverse training datasets, ensuring representation across gender, race, and disability. Additionally, AI models must be transparent about their limitations, encouraging critical evaluation by users.
Steps Toward Improvement:
- Expand Training Datasets: Include more diverse images of professionals across industries.
- Monitor Outputs: Continuously assess AI-generated content for biases.
- Collaborate with Experts: Work with sociologists and educators to improve representation in AI tools.
For real-world applications of generative AI in advertising, read about Coca-Cola’s AI holiday ads.
The Bigger Picture
As generative AI becomes increasingly integrated into education and media, its influence on societal norms cannot be ignored. Tools that fail to reflect diversity may unintentionally perpetuate harmful stereotypes. By addressing these biases, AI developers can ensure that technology serves as a tool for inclusion, inspiring the next generation of scientists, chemists, and innovators.
Explore how women are leading the growth of generative AI in various industries.
Conclusion
The study on AI-generated images of chemists reveals a critical gap in representation, particularly regarding race and disabilities. While some models align with current gender demographics, the overall lack of inclusivity underscores the need for more thoughtful AI development. As we increasingly rely on these tools, addressing biases is essential to ensure they reflect—and celebrate—the diversity of real-world professionals.
For more details, visit TechXplore’s report on this groundbreaking study.
By tackling these challenges, AI can move closer to its potential as an unbiased, empowering tool for all.